Our rationale is that effective integration of proteomics data into a genomic framework will lead to improved knowledge of complex biological systems and facilitate access to protein level data (Paik YK. et. al., Nat Biotechnology, 30(3), 221-223, 2012).
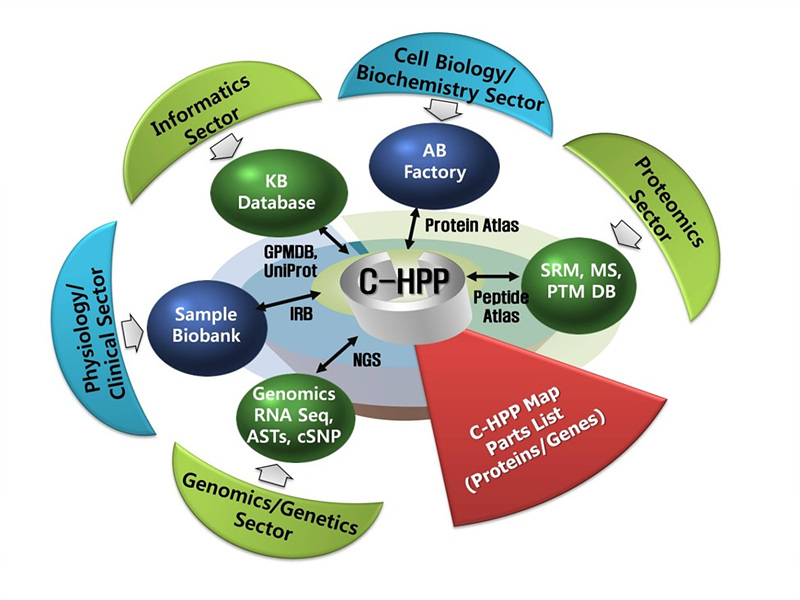
< Components of the C-HPP research module, (Paik YK. et. al., Nat Biotechnology, 30(3), 221-223, 2012) >
With the generation of a well characterized human genome map together with the availability of in depth transcriptomics, the genesis of C-HPP came from the realization that the proteomic community was well placed to study the full complexity of human proteome. Examples of incomplete proteome information include uncharacterized products for known protein coding genes, variants generated by alternative splicing and coding SNPs (cSNPs) (Li et al., Science, 333(6038), 53-8, 2011), and a comprehensive characterization of major post-translational modifications (PTMs). We believe that such a large-scale project will greatly expand our knowledge of the phenotypic state with commensurate payouts in biological and clinical research, such as novel drug targets, molecular diagnostics and therapeutic monitoring (personalized medicine) (Nat Methods. 7, 661, 2010). C-HPP has as a primary goal that the proteomic catalog should be put in the context of the chromosomal gene sequences to promote more effective collaborations with molecular biologists and to improve understanding of the biological context of proteomics data sets (Hancock W. et al., J Proteome Res. 10(1), 210, 2011). The C-HPP was proposed by Young-Ki Paik and his colleagues at several HUPO meetings (Amsterdam, August, 2008; Toronto, September, 2009; Sydney, September, 2010).
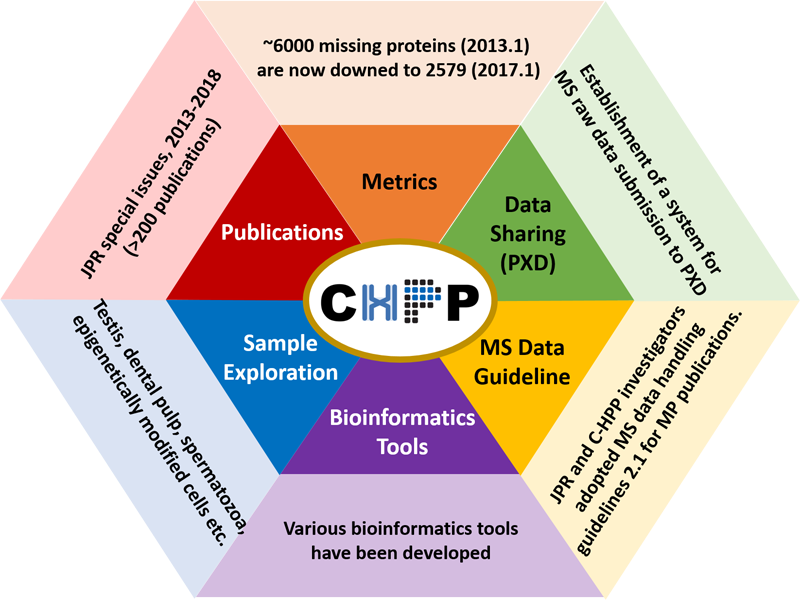
The C-HPP teams have played a key role in setting some HPP milestones in six areas of cooperation with the bioinformatics teams and individual investigators. The areas of cooperation are: (i) the 'Metrics' system for updating the yearly progress in protein annotation, (ii) the PXD data submission rule, which was a first step toward community-wide data sharing, (iii) the MS data interpretation guidelines v2.1 (the Guidelines v2.1), (iv) data managing bioinformatics tools, (v) collaboration for the JPR special issue publications, and (vi) rare sample utilization for missing proteins detection (from Paik et al., Expert Rev Proteomics. 2017 Dec;14(12):1059-107)